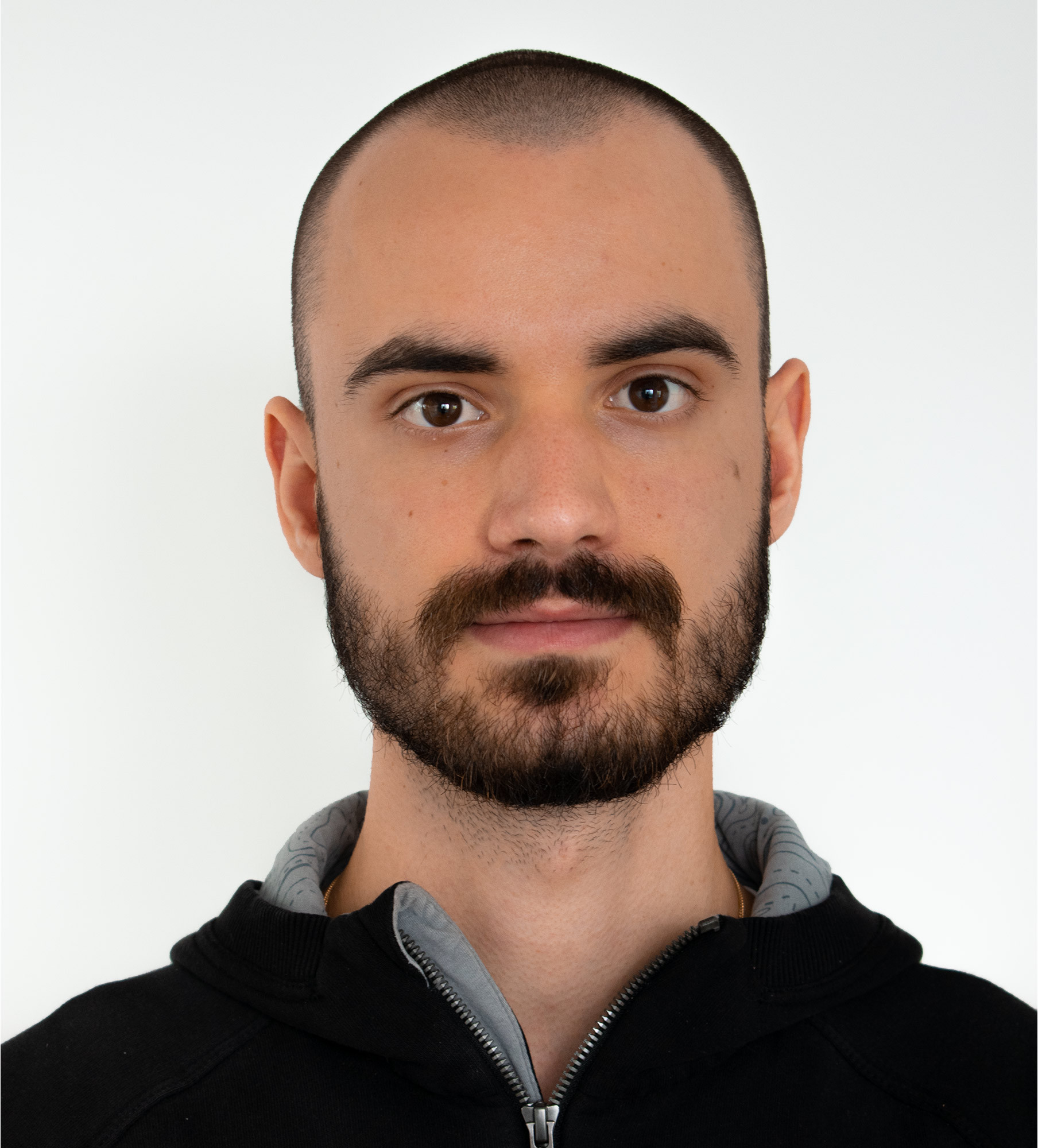
Willi Menapace
Welcome! I am a Ph.D Student at the University of Trento where I work with Elisa Ricci and Nicu Sebe at the Multimedia and Human Understanding Group MHUG. My research interests include the application of deep learning techniques to the computer vision field, in particular in the areas of image and video generation. During my studies I had the pleasure to work with Sergey Tulyakov at Snap Inc., Stéphane Lathuilière at Telecom Paris, Vladislav Golyanik and Christian Theobalt at the Max Planck Institute for Informatics.
Github LinkedIn Google Scholar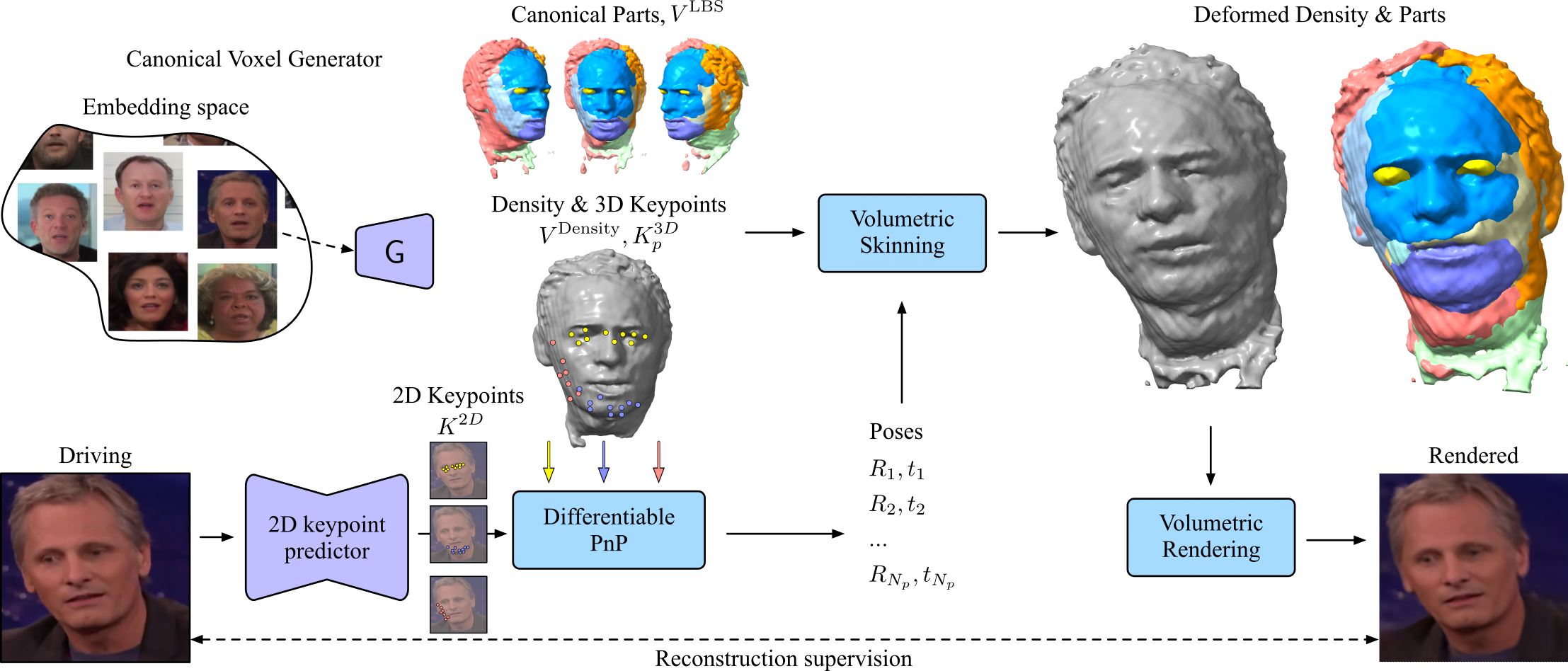
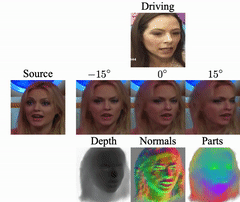
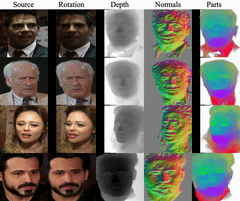
Unsupervised Volumetric Animation
Aliaksandr Siarohin, Willi Menapace, Ivan Skorokhodov, Kyle Olszewski, Hsin-Ying Lee, Jian Ren, Menglei Chai, Sergey Tulyakov
CVPR 2023
We propose a novel approach for unsupervised 3D animation of non-rigid deformable objects. Our method learns the 3D structure and dynamics of objects solely from single-view RGB videos, and can decompose them into semantically meaningful parts that can be tracked and animated. Using a 3D autodecoder framework, paired with a keypoint estimator via a differentiable PnP algorithm, our model learns the underlying object geometry and parts decomposition in an entirely unsupervised manner. This allows it to perform 3D segmentation, 3D keypoint estimation, novel view synthesis, and animation. ...
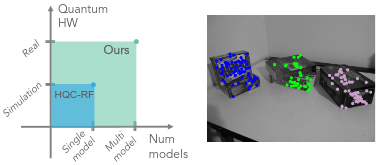
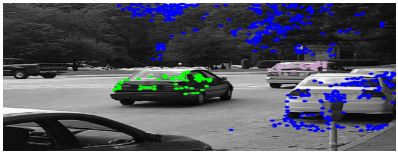
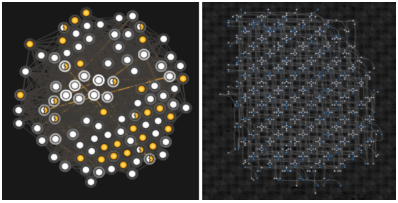
Quantum Multi-Model Fitting
Matteo Farina, Luca Magri, Willi Menapace, Elisa Ricci, Vladislav Golyanik, Federica Arrigoni
CVPR 2023 (Highlight)
Geometric model fitting is a challenging but fundamental computer vision problem. Recently, quantum optimization has been shown to enhance robust fitting for the case of a single model, while leaving the question of multi-model fitting open. In response to this challenge, this paper shows that the latter case can significantly benefit from quantum hardware and proposes the first quantum approach to multi-model fitting (MMF). We formulate MMF as a problem that can be efficiently sampled by modern adiabatic quantum computers without the relaxation of the objective function. We also propose an iterative and decomposed version of our method, which supports real-world-sized problems. The experimental evaluation demonstrates promising results on a variety of datasets.
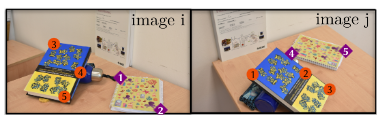
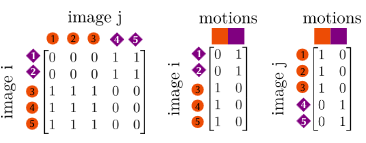
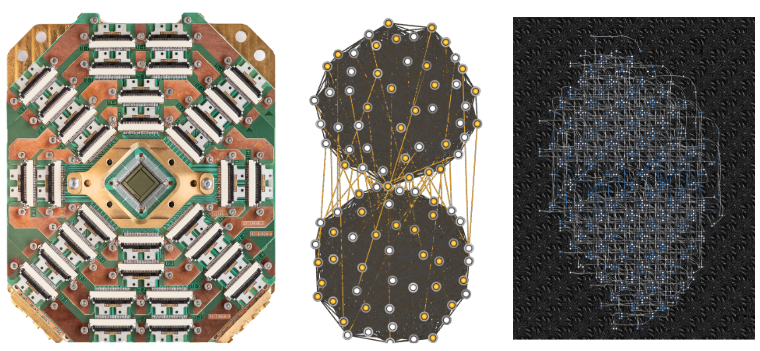
Quantum Motion Segmentation
Federica Arrigoni, Willi Menapace, Marcel Seelbach Benkner, Elisa Ricci, Vladislav Golyanik
ECCV 2022
Motion segmentation is a challenging problem that seeks to identify independent motions in two or several input images. This paper introduces the first algorithm for motion segmentation that relies on adiabatic quantum optimization of the objective function. The proposed method achieves on-par performance with the state of the art on problem instances which can be mapped to modern quantum annealers.
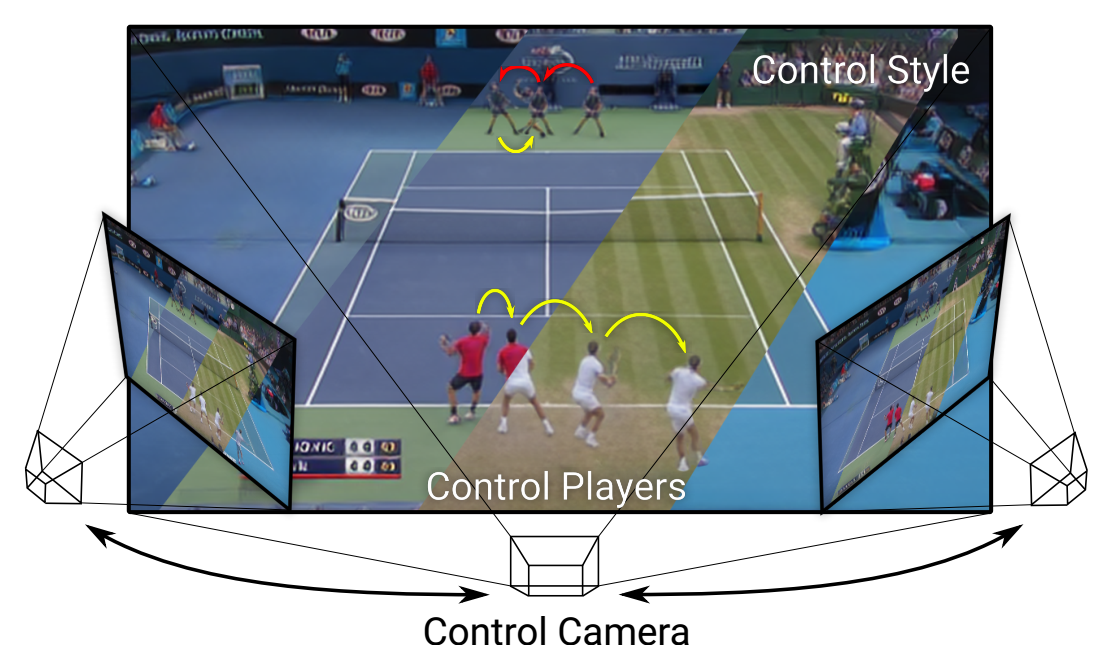
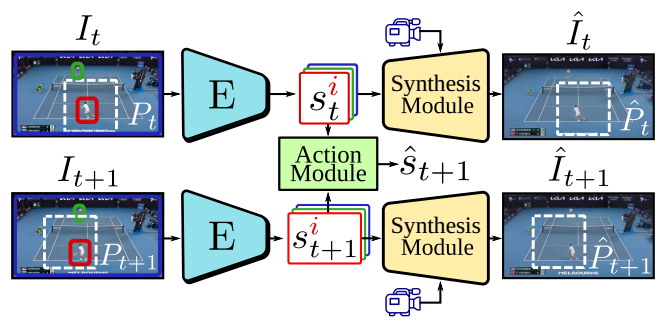
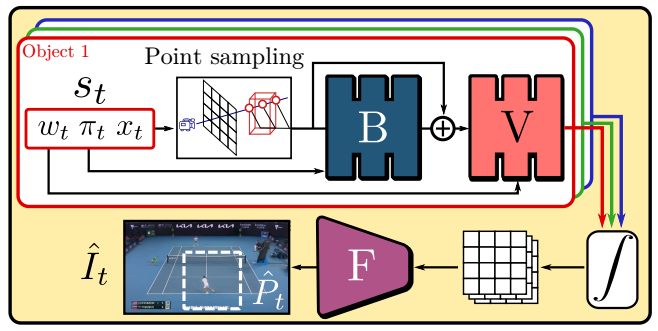
Playable Environments: Video Manipulation in Space and Time
Willi Menapace, Stéphane Lathuilière, Aliaksandr Siarohin, Christian Theobalt, Sergey Tulyakov, Vladislav Golyanik, Elisa Ricci
CVPR 2022
We present Playable Environments - a new representation for interactive video generation and manipulation in space and time. With a single image at inference time, our novel framework allows the user to move objects in 3D while generating a video by providing a sequence of desired actions. The actions are learnt in an unsupervised manner. The camera can be controlled to get the desired viewpoint. Our method builds an environment state for each frame, which can be manipulated by our proposed action module and decoded back to the image space with volumetric rendering. To support diverse appearances of objects, we extend neural radiance fields ...
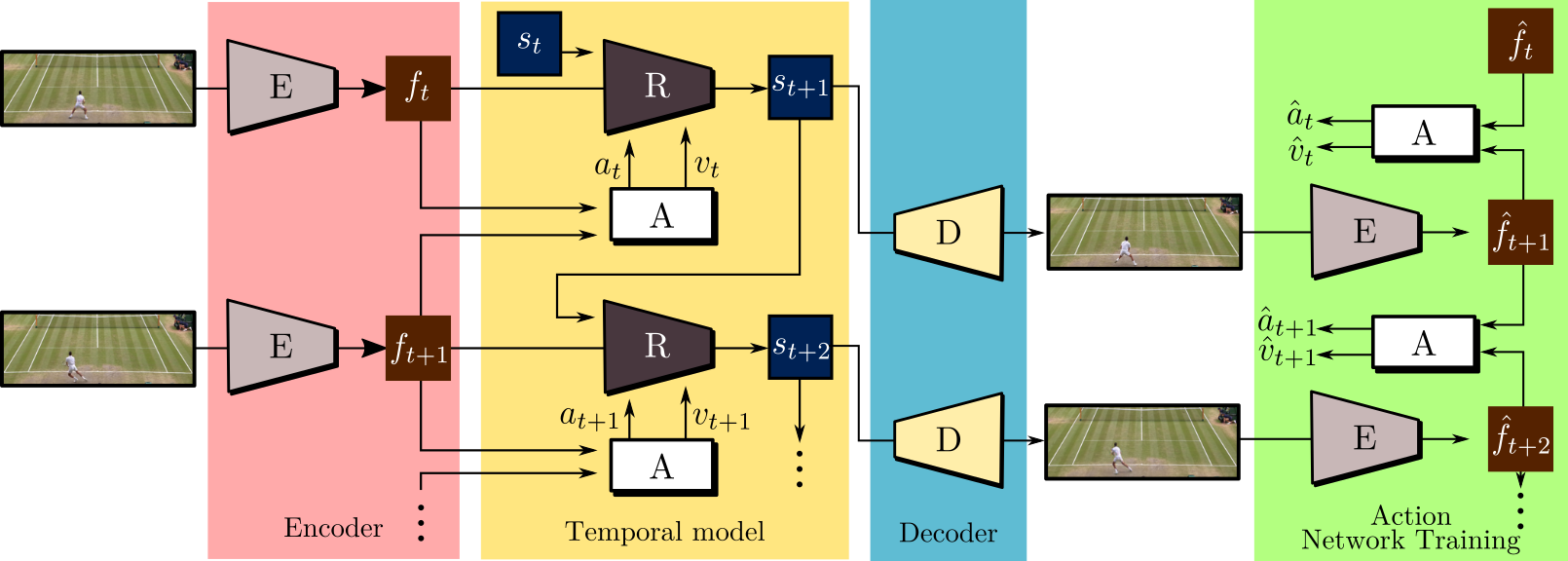
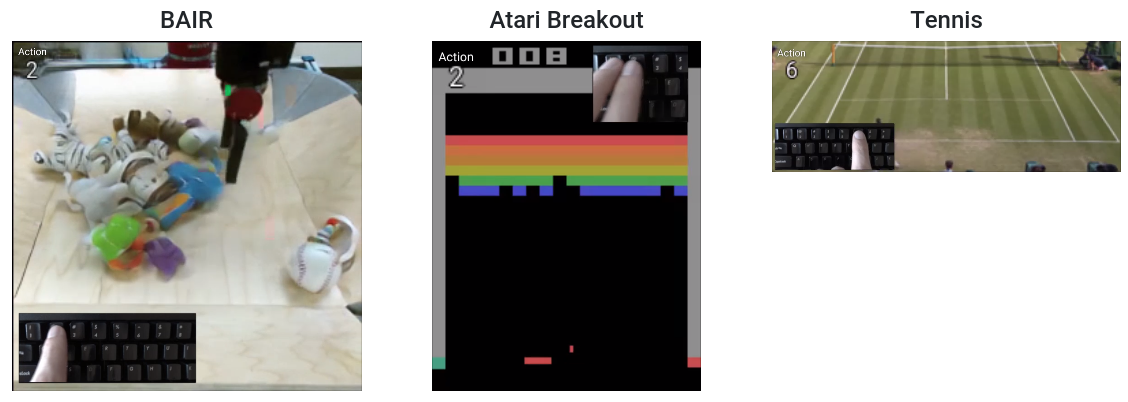
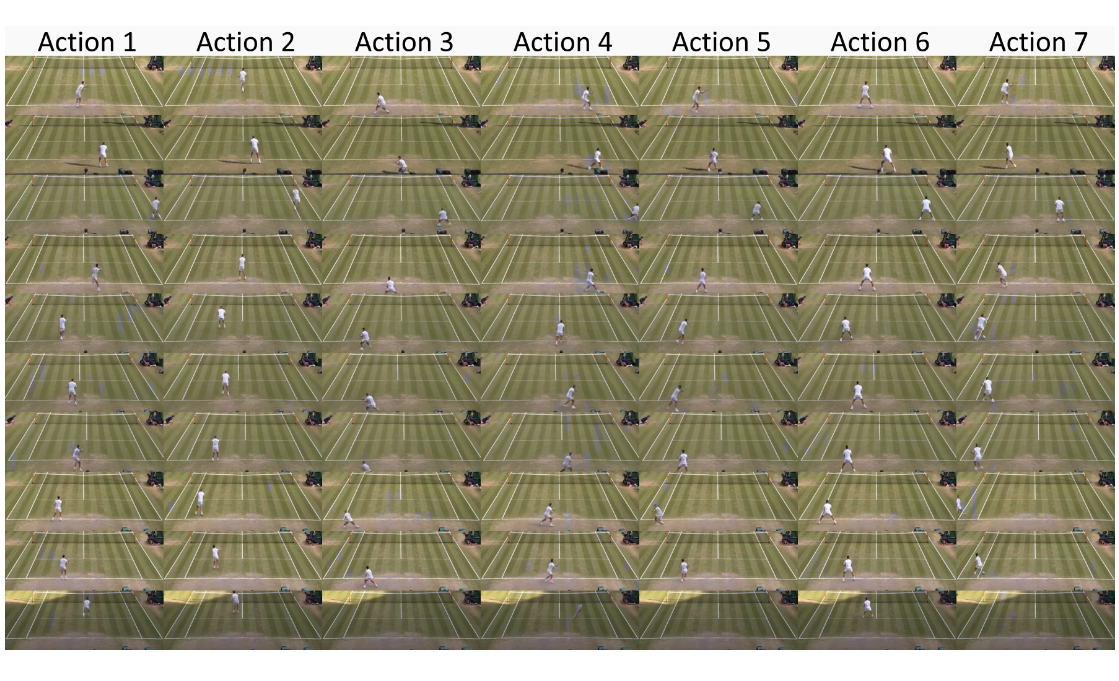
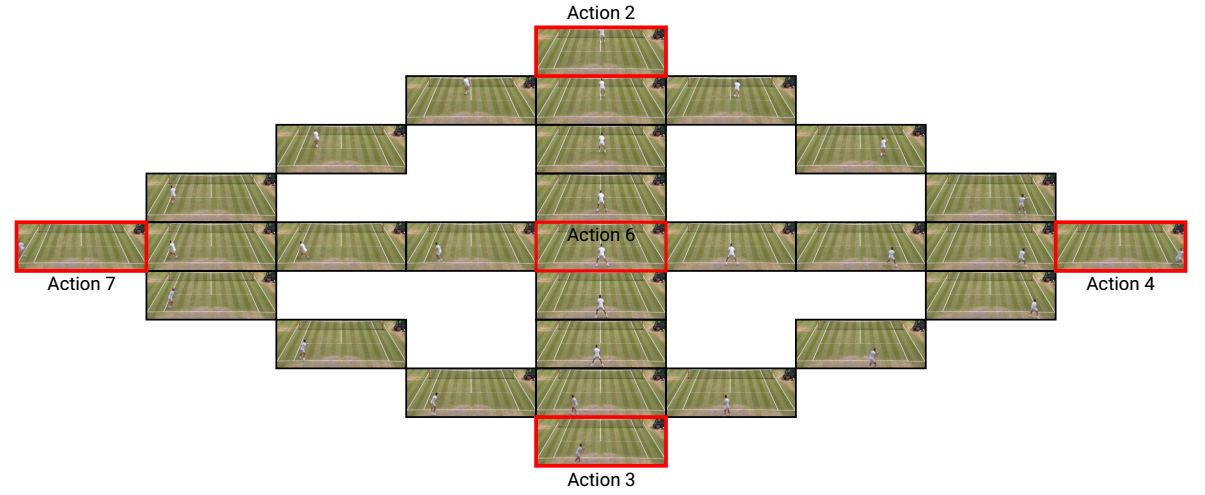
Playable Video Generation
Willi Menapace, Stéphane Lathuilière, Sergey Tulyakov, Aliaksandr Siarohin, Elisa Ricci
CVPR 2021 (Oral)
This paper introduces the unsupervised learning problem of playable video generation (PVG). In PVG, we aim at allowing a user to control the generated video by selecting a discrete action at every time step as when playing a videogame. The difficulty of the task lies both in learning semantically consistent actions and in generating realistic videos conditioned on the user input. We propose a novel framework for PVG that is trained in a self-supervised manner on a large dataset of unlabelled videos. We employ an encoder-decoder architecture where the predicted action labels act as ...
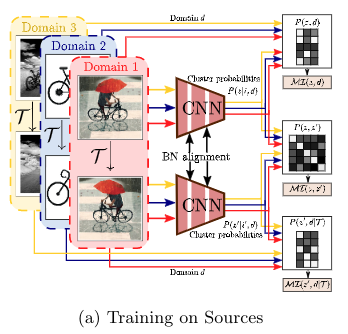
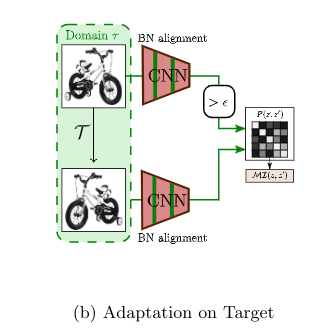
Learning to Cluster under Domain Shift
Willi Menapace, Stéphane Lathuilière, Elisa Ricci
ECCV 2020
While unsupervised domain adaptation methods based on deep architectures have achieved remarkable success in many computer vision tasks, they rely on a strong assumption, i.e. labeled source data must be available. In this work we overcome this assumption and we address the problem of transferring knowledge from a source to a target domain when both source and target data have no annotations. Inspired by recent works on deep clustering, our approach leverages information from data gathered from multiple source domains to build a domain-agnostic clustering model which is then refined at inference time when target data become available. ...
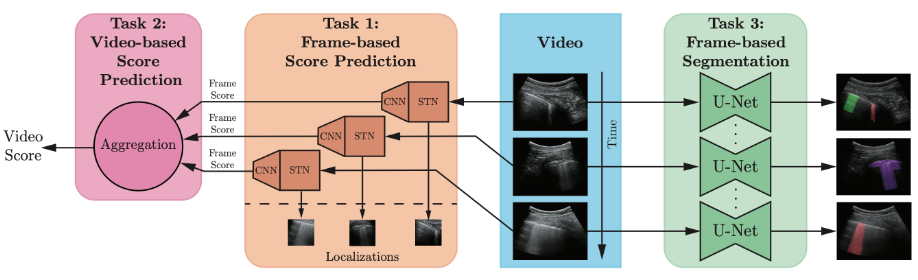
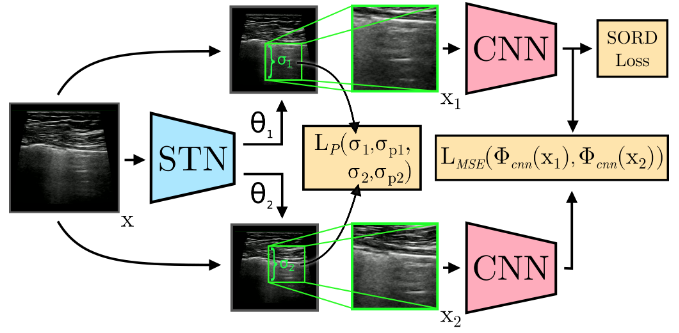
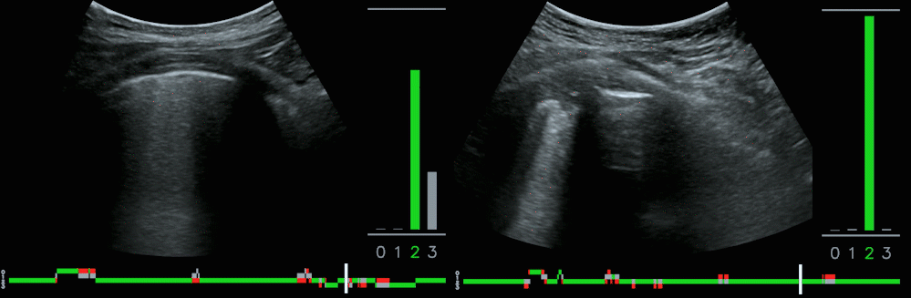
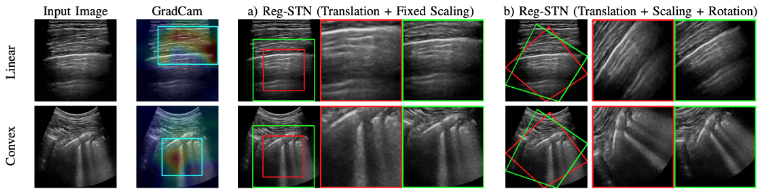
Deep Learning for Classification and Localization of COVID-19 Markers in Point-of-Care Lung Ultrasound
Subhankar Roy*, Willi Menapace*, Sebastiaan Oei, et al.
IEEE Transactions on Medical Imaging
Deep learning (DL) has proved successful inmedical imaging and, in the wake of the recent COVID-19 pandemic, some works have started to investigate DL-based solutions for the assisted diagnosis of lung diseases. While existing works focus on CT scans, this paper studies the application of DL techniques for the analysisof lung ultrasonography (LUS) images. Specifically, we present a novel fully-annotated dataset of LUS images collected from several Italian hospitals, with labels indicating the degree of disease severity at a frame-level, video-level, and pixel-level (segmentation masks). ...
- ICCV 2023
- CVPR 2023
- ACM MultiMedia 2023
- WACV 2023
- CVPR 2022
- CVPR MULA 2022
- CVPR MULA 2021
- ACM MultiMedia 2021
- ICRA 2021
- ACM MultiMedia 2020
- Spring - Winter 2023: Research Intern at Snap Inc. supervised by Sergey Tulyakov, Aliaksandr Siarohin
- Summer 2022: Research Intern at Snap Inc. supervised by Sergey Tulyakov, Aliaksandr Siarohin
- Spring 2021 - Spring 2022: Research Intern at Max Planck Institute for Informatics supervised by Christian Theobalt, Vladislav Golyanik
- Summer 2019: Intern, Deep Learning at eXact lab
- Spring 2019: External Collaborator, Deep Learning at eXact lab
- Summer 2017: Intern, CUDA/OpenCL Developer at eXact lab
- Summer 2014: Intern, Data Analyst/C# Programmer at Famas System
- Summer 2013: Intern, Software Engineering at FBK